Hyperspectral imaging (HSI) is a cutting-edge imaging technology which allows for accurate material identification. HSI can make use of visible, infrared and ultraviolet light and in addition to modalities such as Raman spectroscopy, give rise to a myriad of interesting applications. Some of these include quality control on various food, detection of defects in materials, sorting of visually similar objects as well as advanced remote sensing and microscopy applications.

A regular RGB camera, just like the human eye, gathers information in three color bands: red, green and blue. A hyperspectral camera can divide the light spectrum into many more bands, which allows for chemical identification using the light reflected from a material. Hyperspectral imaging is therefore far more powerful in the classification of materials. When compared to a standard spectrometer, a hyperspectral camera has the advantage of not only using spectral, but also spatial information. A hyperspectral camera takes images with detailed light information for each pixel, while a classical spectrometer can be seen as a hyperspectral camera with only one pixel.
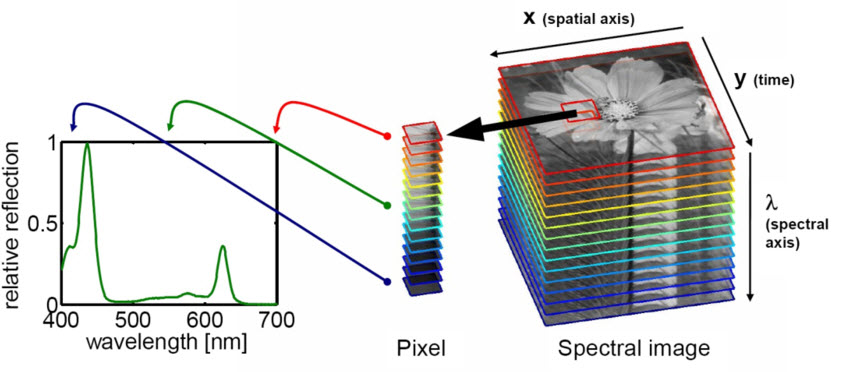
Hyperspectral imaging and deep learning
Hyperspectral imaging synergizes very nicely with deep learning and machine learning. Interesting patterns can be found in the detailed spectral information which are often not available in more common RGB imaging. In hyperspectral classification, halogen or specialized LED illumination is needed to cover a wide spectrum while environmental light and reflections should be limited. Multiple hyperspectral cameras can be used to increase the spectral range.
Widespread use in a range of areas
Hyperspectral imaging finds applications in a range of areas, including the following:
- Quality control (e.g. the rejection of spoiled foods or the inspection of material quality)
- Crop inspection (e.g. identify mildew infected crops to avoid large crop losses on plantations)
- Sorting machines (e.g. fabrics/textiles, recycling, food, construction materials)
- Moisture detection
- Obtaining mineral composition in geological samples
- Biomedical
- Remote sensing
- Surveillance
- Astronomy
Numerous practical examples illustrate HSI enabled applications. A first example addresses the detection of mildew on a variety of fruits and vegetables, which is critical to prevent the spread of this crop disease. Drones equipped with hyperspectral cameras identify infected crops in an early stage so that prompt corrective action can be taken. This avoids large crop losses on plantations with lettuce, beets, spinach, grapevines, etc.
Another typical application is found in plastic recycling. The process starts from small shredded plastic fragments transported on a conveyor belt. Hyperspectral cameras are used to identify the main material each fragment is made of. Robust material-based sorting is essential in correctly feeding multiple parallel recycling flows.
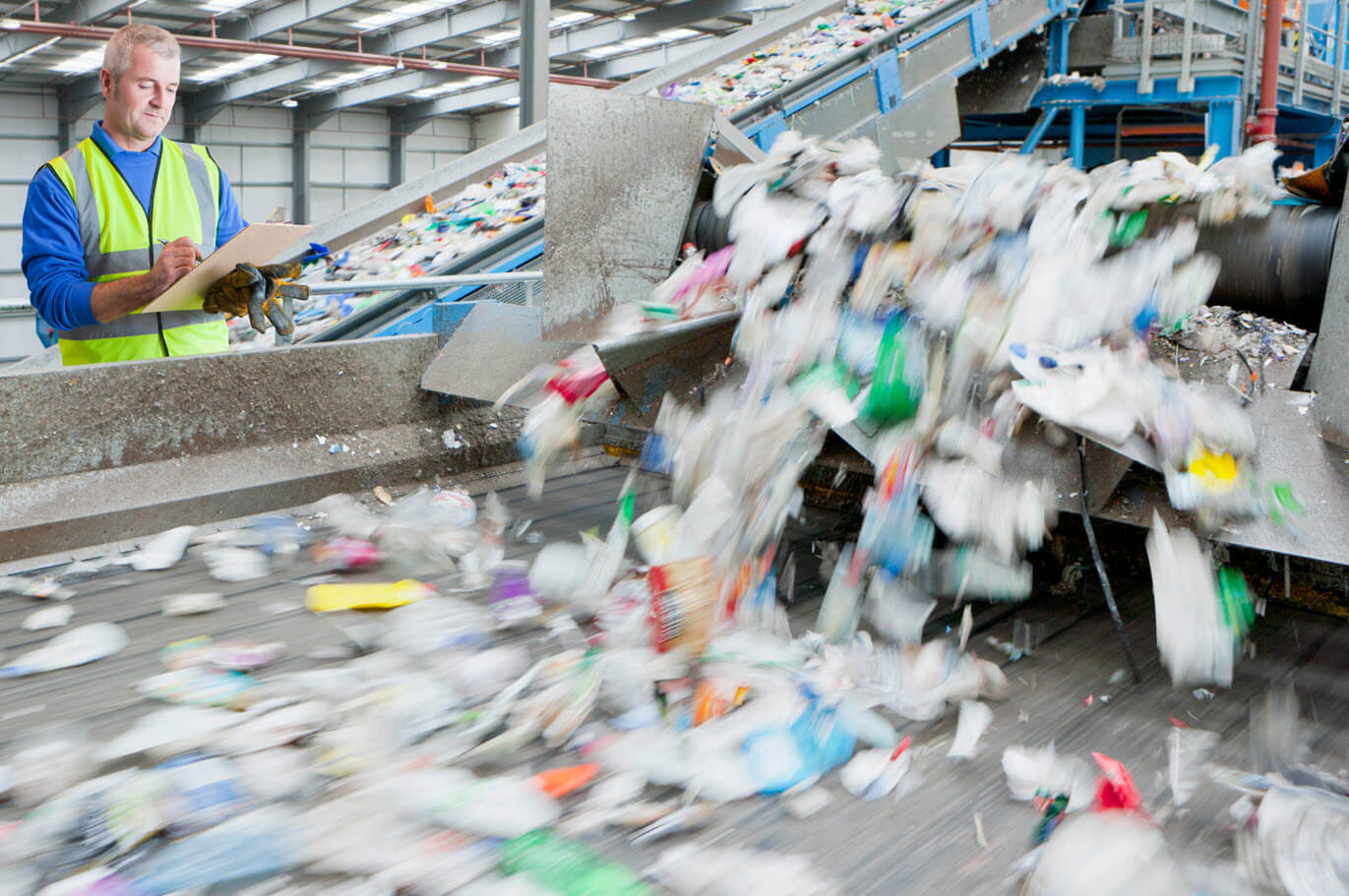
Example application: Grading Scanner
In the dynamic landscape of food production, where standards and expectations continually evolve, quality control and grading play a pivotal role. Ensuring that products meet strict quality standards is essential for both consumer safety and the success of food-related businesses. However, human interpretation can introduce inaccuracies during the grading and quality control processes, potentially leading to inconsistencies in product quality and safety. It's in such scenarios that automation and technology become invaluable, offering a more consistent and objective analysis of food products.
To streamline the grading process and enhance accuracy, we have developed the Hyperspectral Grading Scanner. Our state-of-the-art, comprehensive grading device leverages hyperspectral imaging technology and deep learning algorithms for automatic grading and defect detection.
To learn more about the Hyperspectral Grading Scanner, click here.
Is HSI suitable for your application?
However, hyperspectral imaging is not always the most suitable technology for your specific application. Sometimes it is better to use regular RGB or even monochrome imaging. HSI has the potential for higher classification accuracies than these modalities, however there are also drawbacks. Hyperspectral imaging is generally slower than RGB/monochrome imaging, generates substantially more data, demands more computational power, and requires stable lighting to be viable. In addition, because the generated data are more complex, more development work is required. HSI is valuable in many applications, but it is important to take these points into consideration. APIXA will gladly help you select the right technology for your specific application challenges.
We suggest hyperspectral imaging when your problem demands:
- Separation of materials which are very similar in color
- Very accurate identification of materials
We would advise against hyperspectral imaging when your application requires:
- High sample throughput
- Low cost
- Short development time
- Environment where control of lighting is difficult